Machine Learning (ML) and Artificial Intelligence (AI) are common buzzwords for IT professionals today, but when applied to your organization's Data Centers, they offer real potential to help your Business grow sustainably.
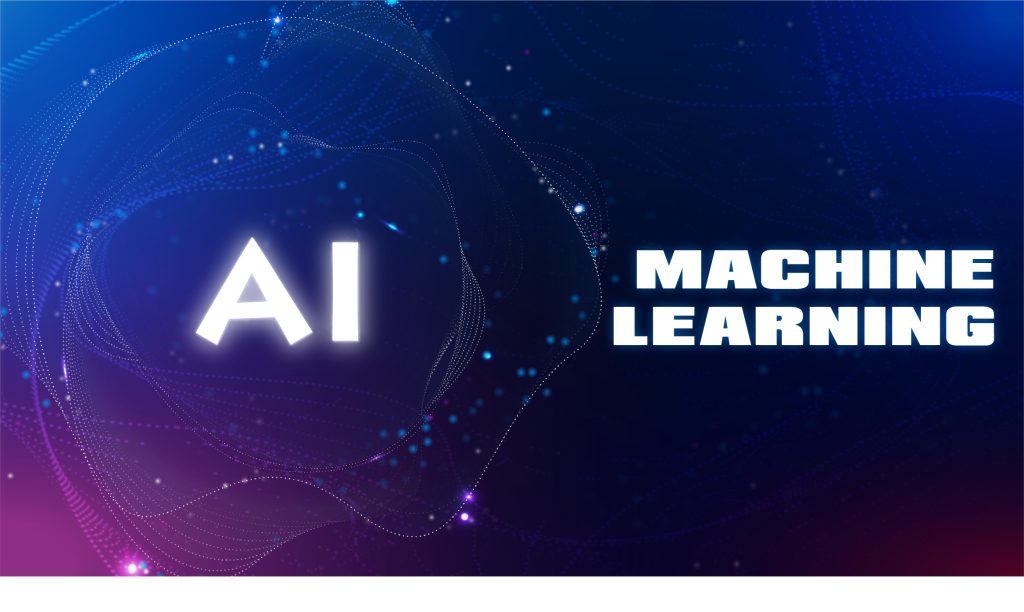
Machine learning software can proactively predict situations faster than you or your team can perceive, and can even resolve problems faster. These systems are a logical extension of today's hybrid Data Center environment and are increasingly becoming a critical part of the Data Center infrastructure.
According to a 2022 Uptime Institute survey, 57% of data center operators trust AI and ML to make routine operational decisions; however, many still only use them for power optimization, cooling, and anomaly detection.
73% of Uptime Institute 2023 survey respondents believe that AI-based software will reduce on-site staffing, and 25% of those believe this will happen within five years. Artificial intelligence and machine learning in data centers can optimize many overall operations, including planning and design, workloads, uptime, and cost management.
ADVANTAGES OF APPLYING MACHINE LEARNING SOFTWARE TO DATA CENTERS
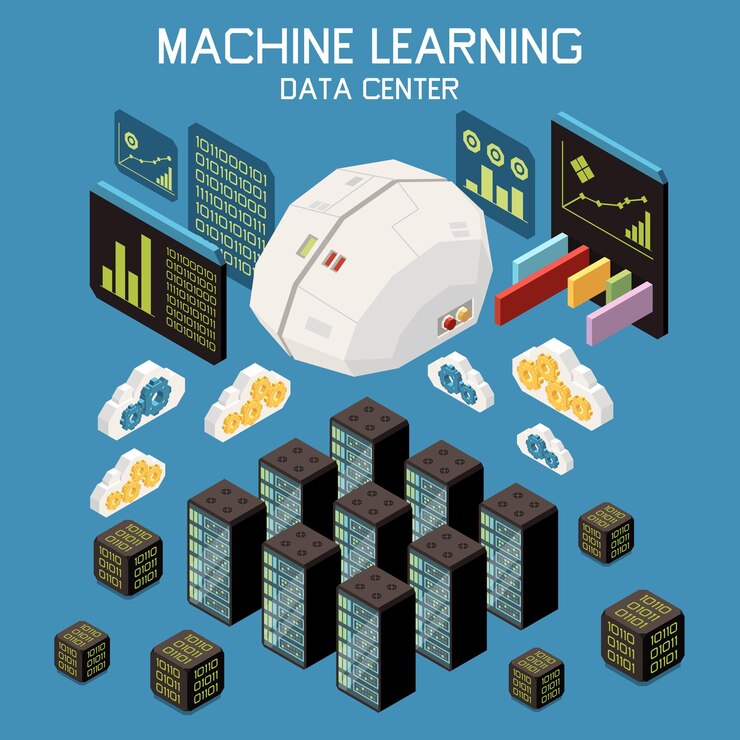
Machine learning can learn from scenarios and data sets to respond instantly instead of requiring human intervention or relying on a pre-programmed set of actions. This technology helps you better understand your data center systems, manage them more efficiently, and prevent unplanned downtime. Here are some use cases for Machine Learning and AI to optimize data center performance:
Create More Efficient Data Centers
Companies can use Machine Learning to automatically manage the physical environment of their Data Centers with software-driven real-time adjustments to the Data Center infrastructure and architecture, rather than just issuing software alerts.
Google, Meta, and Microsoft use artificial intelligence and machine learning to monitor energy optimization and performance in their Data Centers.
Reducing Operational Risk
Preventing downtime is critical to Data Center operations. Machine Learning can predict and prevent downtime. Data Center Machine Learning software monitors real-time performance data from critical equipment – such as power management and cooling systems – and predicts when hardware is likely to fail. This allows operators to perform preventative maintenance and prevent costly failures.
Machine Learning-based risk analytics improves Data Center uptime by modeling different configurations to increase resiliency; identifying preventative maintenance opportunities; and detecting potential cybersecurity risks before they occur.
Reduce customer churn with data intelligence
Companies can use Machine Learning in the Data Center to better understand customers and predict their behavior. As an extension of customer success programs, Machine Learning can analyze large volumes of information in Data Centers that often go unused after collection.
By connecting Machine Learning software to a customer relationship management (CRM) system, an AI-powered Data Hub can search and retrieve data stored in historical databases, which is not used for traditional CRM. This allows the CRM system to build different strategies to grow or improve customer success.
Energy Consumption Management
Organizations can manage energy with Machine Learning and see significant benefits immediately. For example, Siemens' Datacenter Clarity LC data center infrastructure management software uses AI-powered tools to analyze each server to detect anomalies and optimization opportunities.
Machine Learning and AI tools detect and redirect workloads from underperforming servers to more energy-efficient and workload-efficient servers with lower utilization rates. Operators receive notifications to replace aging servers, giving them time to upgrade before servers become obsolete.
Analyzing System Logs
Most Data Center systems generate logs during operation, but they are useless if not managed. Machine Learning can centralize and analyze logs, creating easy-to-use reports for data center teams. Open source technologies like Elasticsearch and paid solutions from Splunk can analyze and process the data collected by Machine Learning processes.
Perform Root Cause Analysis.
When performance failures occur, operators must quickly identify the root cause and fix it. AI-powered predictive tools, such as Hewlett Packard Enterprise’s InfoSight, provide the tools to identify and resolve failures in near real-time across an organization’s on-premises and cloud data centers.
Source: https://www.techtarget.com